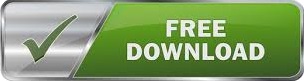
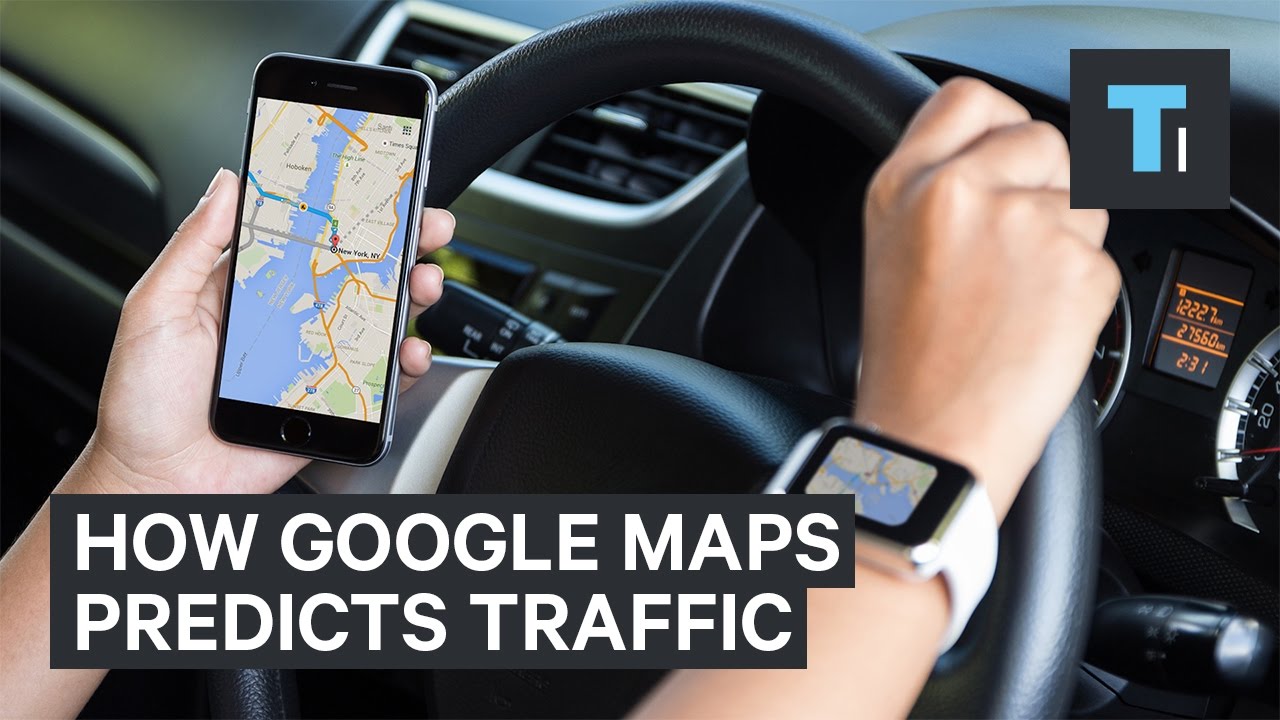
On the road to novel machine learning architectures for traffic prediction The model architecture for determining optimal routes and their travel time. Currently, the Google Maps traffic prediction system consists of the following components: (1) a route analyser that processes terabytes of traffic information to construct Supersegments and (2) a novel Graph Neural Network model, which is optimised with multiple objectives and predicts the travel time for each Supersegment. We divided road networks into “Supersegments” consisting of multiple adjacent segments of road that share significant traffic volume. Here’s how it works: Dividing the world’s roads into Supersegments To do this at a global scale, we used a generalised machine learning architecture called Graph Neural Networks that allows us to conduct spatiotemporal reasoning by incorporating relational learning biases to model the connectivity structure of real-world road networks. While Google Maps’ predictive ETAs have been consistently accurate for over 97% of trips, we worked with the team to minimise the remaining inaccuracies even further - sometimes by more than 50% in cities like Taichung. Additional factors like road quality, speed limits, accidents, and closures can also add to the complexity of the prediction model.ĭeepMind partnered with Google Maps to help improve the accuracy of their ETAs around the world. For example - even though rush-hour inevitably happens every morning and evening, the exact time of rush hour can vary significantly from day to day and month to month. This process is complex for a number of reasons. To accurately predict future traffic, Google Maps uses machine learning to combine live traffic conditions with historical traffic patterns for roads worldwide.
#Future traffic google maps driver#
While this data gives Google Maps an accurate picture of current traffic, it doesn’t account for the traffic a driver can expect to see 10, 20, or even 50 minutes into their drive. To calculate ETAs, Google Maps analyses live traffic data for road segments around the world.
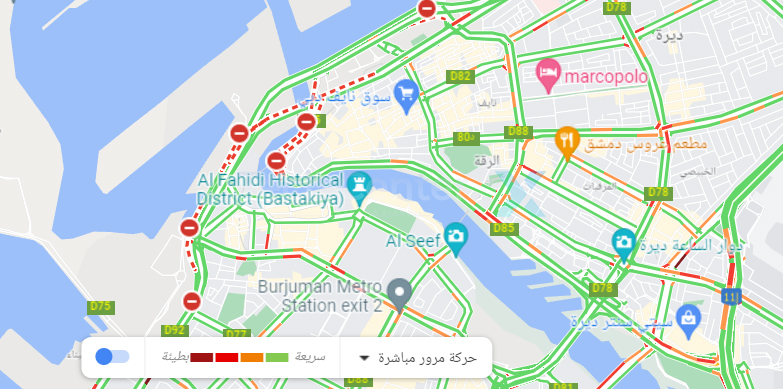
by using advanced machine learning techniques including Graph Neural Networks, as the graphic below shows:
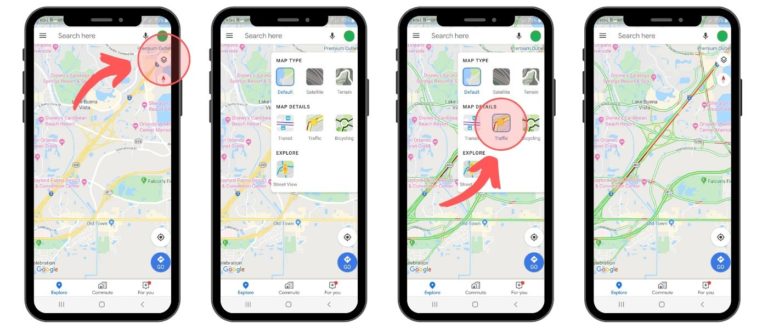
Researchers at DeepMind have partnered with the Google Maps team to improve the accuracy of real time ETAs by up to 50% in places like Berlin, Jakarta, São Paulo, Sydney, Tokyo, and Washington D.C. These features are also useful for businesses such as rideshare companies, which use Google Maps Platform to power their services with information about pickup and dropoff times, along with estimated prices based on trip duration.

These are critical tools that are especially useful when you need to be routed around a traffic jam, if you need to notify friends and family that you’re running late, or if you need to leave in time to attend an important meeting. People rely on Google Maps for accurate traffic predictions and estimated times of arrival (ETAs). Today we’re delighted to share the results of our latest partnership, delivering a truly global impact for the more than one billion people that use Google Maps. From reuniting a speech-impaired user with his original voice, to helping users discover personalised apps, we can apply breakthrough research to immediate real-world problems at a Google scale. By partnering with Google, DeepMind is able to bring the benefits of AI to billions of people all over the world.
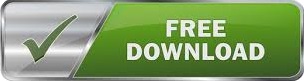